Autonomous driving technology is revolutionizing the transportation industry, and visual point cloud forecasting is at the forefront of this transformation. As vehicles become smarter and more autonomous, the need for precise and scalable forecasting systems has never been greater. This breakthrough technology allows vehicles to predict and respond to their surroundings with unparalleled accuracy, paving the way for safer and more efficient autonomous driving.
The future of autonomous driving depends heavily on the ability to process vast amounts of data in real time. Visual point cloud forecasting leverages advanced algorithms and machine learning techniques to create detailed 3D models of the environment, enabling vehicles to anticipate potential hazards and make informed decisions. This innovation is not only crucial for the development of self-driving cars but also for enhancing the overall safety and reliability of autonomous systems.
In this article, we will delve into the intricacies of visual point cloud forecasting, exploring its applications, challenges, and potential to revolutionize autonomous driving. By understanding the technology behind this forecasting system, we can gain insights into how it is shaping the future of transportation and mobility.
Read also:Ernies Market Oak Park Mi Your Ultimate Guide To Shopping And Community
What is Visual Point Cloud Forecasting?
Visual point cloud forecasting is a cutting-edge technology that uses sensors and cameras to capture and analyze 3D data from the surrounding environment. This data is then processed to create detailed point clouds, which are essentially collections of data points representing the surfaces of objects in the environment. These point clouds are used to forecast future scenarios, allowing autonomous vehicles to anticipate changes in their surroundings.
One of the key advantages of visual point cloud forecasting is its ability to scale effectively. By leveraging advanced computational techniques, this technology can handle large datasets and provide accurate predictions even in complex urban environments. This scalability makes it an ideal solution for autonomous driving, where vehicles must navigate through a variety of conditions and scenarios.
Applications of Visual Point Cloud Forecasting in Autonomous Driving
Predicting Vehicle Behavior
One of the primary applications of visual point cloud forecasting is in predicting the behavior of other vehicles on the road. By analyzing the movement patterns of nearby cars, autonomous vehicles can anticipate potential hazards and adjust their driving accordingly. This predictive capability is essential for ensuring safe and efficient navigation in dynamic traffic environments.
Object Detection and Classification
Visual point cloud forecasting also plays a critical role in object detection and classification. By identifying and categorizing objects such as pedestrians, cyclists, and obstacles, autonomous vehicles can make informed decisions about how to interact with their surroundings. This technology enables vehicles to recognize and respond to potential hazards in real time, enhancing overall safety.
Traffic Flow Analysis
Another important application of visual point cloud forecasting is in traffic flow analysis. By analyzing patterns in traffic movement, autonomous vehicles can optimize their routes and reduce congestion. This capability is particularly valuable in urban areas, where traffic conditions can vary significantly throughout the day.
Challenges in Implementing Visual Point Cloud Forecasting
Despite its many advantages, implementing visual point cloud forecasting in autonomous driving systems presents several challenges. One of the primary challenges is ensuring the accuracy and reliability of the forecasting algorithms. As vehicles rely heavily on these predictions to make critical decisions, any errors or inaccuracies can have serious consequences.
Read also:Lexisoriya Leaks Unveiling The Truth Behind The Controversy
Another challenge is managing the computational demands of processing large datasets in real time. Autonomous vehicles must be equipped with powerful hardware and software to handle the complex calculations required for visual point cloud forecasting. This requires significant investment in research and development to optimize both the hardware and algorithms used in these systems.
Advantages of Visual Point Cloud Forecasting
Enhanced Safety
One of the most significant advantages of visual point cloud forecasting is its ability to enhance safety in autonomous driving. By providing accurate predictions of potential hazards, this technology enables vehicles to take preventive actions and avoid accidents. This is particularly important in high-speed scenarios, where reaction times are critical for ensuring safety.
Improved Efficiency
Visual point cloud forecasting also contributes to improved efficiency in autonomous driving systems. By optimizing traffic flow and route planning, vehicles can reduce travel times and fuel consumption. This not only benefits individual drivers but also has a positive impact on the environment by reducing emissions and congestion.
Scalability
One of the standout features of visual point cloud forecasting is its scalability. This technology can be adapted to a wide range of environments and conditions, making it suitable for use in both urban and rural areas. Its ability to handle large datasets and complex scenarios ensures that it remains effective even as the demands of autonomous driving continue to grow.
How Visual Point Cloud Forecasting Works
Data Collection
The process of visual point cloud forecasting begins with data collection. Sensors such as LiDAR, cameras, and radar are used to capture detailed information about the environment. This data is then processed to create point clouds, which serve as the foundation for the forecasting algorithms.
Data Processing
Once the data has been collected, it is processed using advanced algorithms to identify patterns and trends. Machine learning techniques are employed to analyze the data and generate predictions about future scenarios. These predictions are then used to inform the decision-making processes of autonomous vehicles.
Real-Time Decision Making
The final step in visual point cloud forecasting is real-time decision making. Autonomous vehicles use the predictions generated by the forecasting algorithms to make informed decisions about how to navigate their surroundings. This ensures that vehicles can respond quickly and effectively to changing conditions, enhancing both safety and efficiency.
Key Technologies Behind Visual Point Cloud Forecasting
LiDAR
LiDAR (Light Detection and Ranging) is a critical component of visual point cloud forecasting. This technology uses lasers to measure distances and create detailed 3D maps of the environment. LiDAR provides high-resolution data that is essential for accurate forecasting and decision making in autonomous driving systems.
Machine Learning
Machine learning plays a vital role in visual point cloud forecasting by enabling the system to learn from experience and improve over time. By analyzing large datasets and identifying patterns, machine learning algorithms can generate more accurate and reliable predictions. This continuous learning process ensures that autonomous vehicles remain effective in a wide range of scenarios.
Computer Vision
Computer vision is another key technology used in visual point cloud forecasting. By analyzing images captured by cameras, computer vision algorithms can identify objects and track their movement. This capability is essential for detecting and responding to potential hazards in real time.
Future Developments in Visual Point Cloud Forecasting
As research and development in autonomous driving technology continues, we can expect to see significant advancements in visual point cloud forecasting. One area of focus is improving the accuracy and reliability of forecasting algorithms, which will enhance the safety and efficiency of autonomous vehicles. Additionally, efforts are underway to reduce the computational demands of these systems, making them more accessible and cost-effective for widespread use.
Another promising development is the integration of visual point cloud forecasting with other technologies, such as 5G networks and edge computing. These advancements will enable faster and more efficient data processing, further enhancing the capabilities of autonomous driving systems.
Impact of Visual Point Cloud Forecasting on the Transportation Industry
The impact of visual point cloud forecasting on the transportation industry is significant. By enabling safer and more efficient autonomous driving, this technology has the potential to transform the way we travel. It can reduce accidents caused by human error, alleviate traffic congestion, and provide greater accessibility for individuals who are unable to drive themselves.
Furthermore, visual point cloud forecasting can have a positive impact on the environment by reducing emissions and promoting sustainable transportation solutions. As more vehicles adopt this technology, we can expect to see a shift towards smarter, greener, and more connected transportation systems.
Conclusion
Visual point cloud forecasting is a groundbreaking technology that is revolutionizing autonomous driving. By enabling vehicles to predict and respond to their surroundings with unparalleled accuracy, this technology is paving the way for safer and more efficient transportation systems. Its applications in predicting vehicle behavior, object detection, and traffic flow analysis highlight its versatility and potential to transform the industry.
While there are challenges to implementing this technology, ongoing research and development are addressing these issues and driving innovation. As visual point cloud forecasting continues to evolve, its impact on the transportation industry will only grow stronger. We encourage readers to share their thoughts and experiences in the comments section below and explore other articles on our site to learn more about the latest advancements in autonomous driving technology.
Table of Contents
- What is Visual Point Cloud Forecasting?
- Applications of Visual Point Cloud Forecasting in Autonomous Driving
- Challenges in Implementing Visual Point Cloud Forecasting
- Advantages of Visual Point Cloud Forecasting
- How Visual Point Cloud Forecasting Works
- Key Technologies Behind Visual Point Cloud Forecasting
- Future Developments in Visual Point Cloud Forecasting
- Impact of Visual Point Cloud Forecasting on the Transportation Industry
- Conclusion
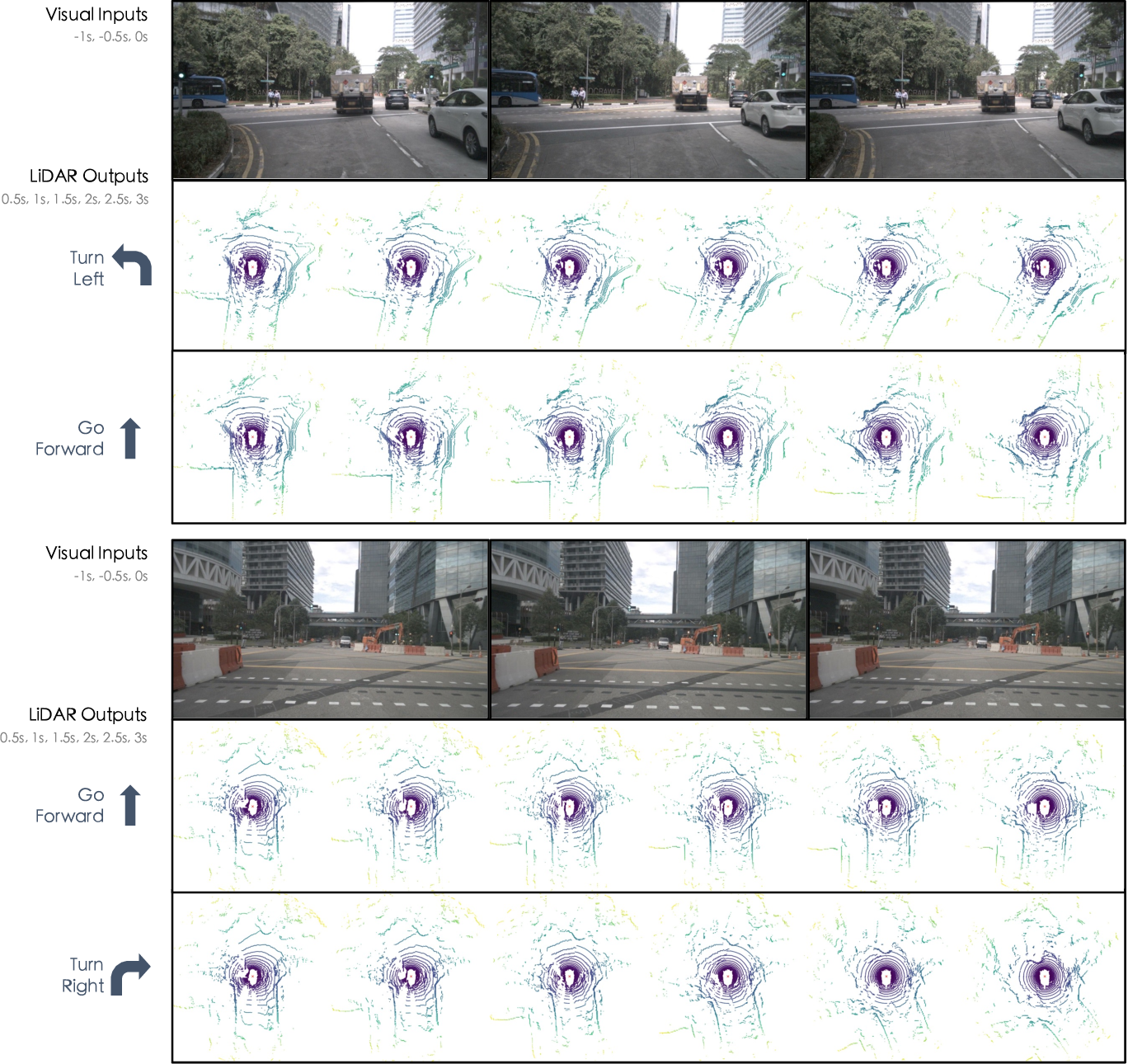
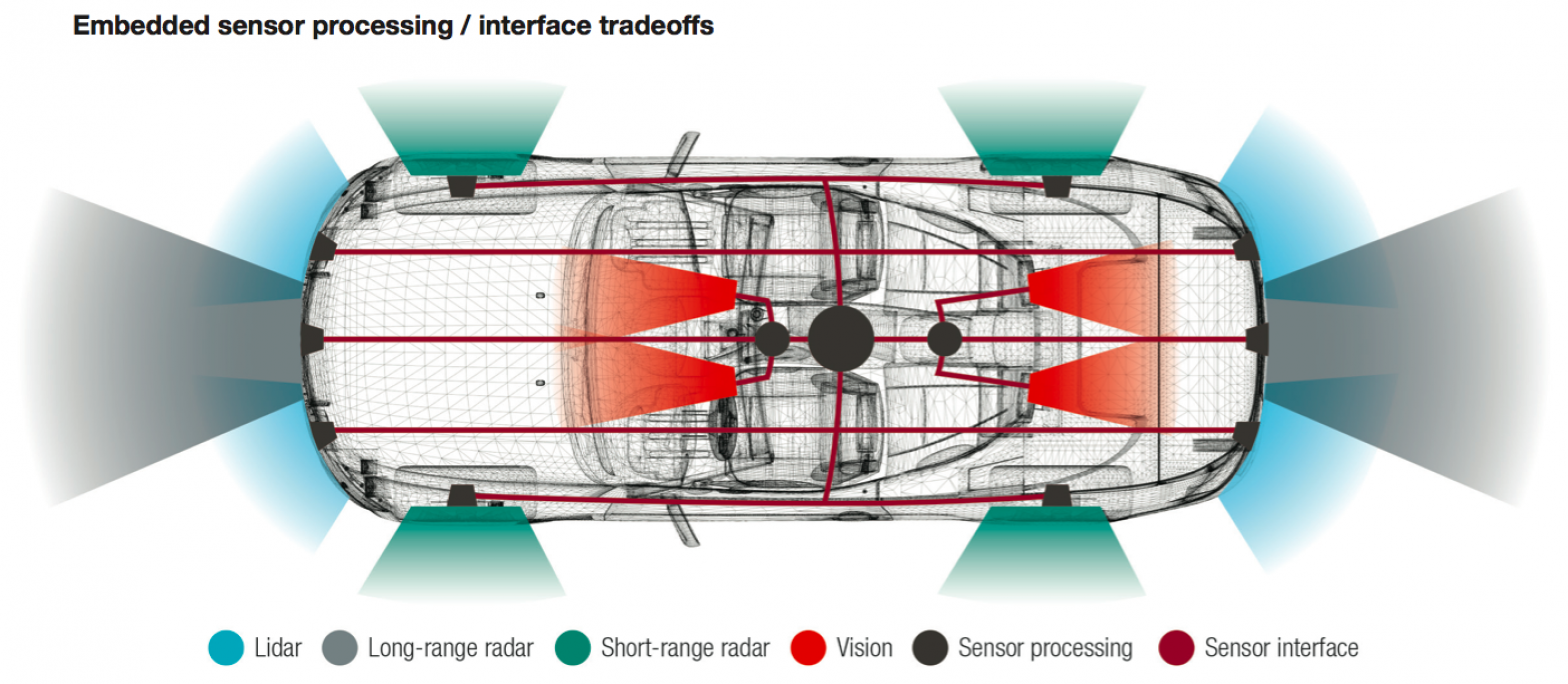
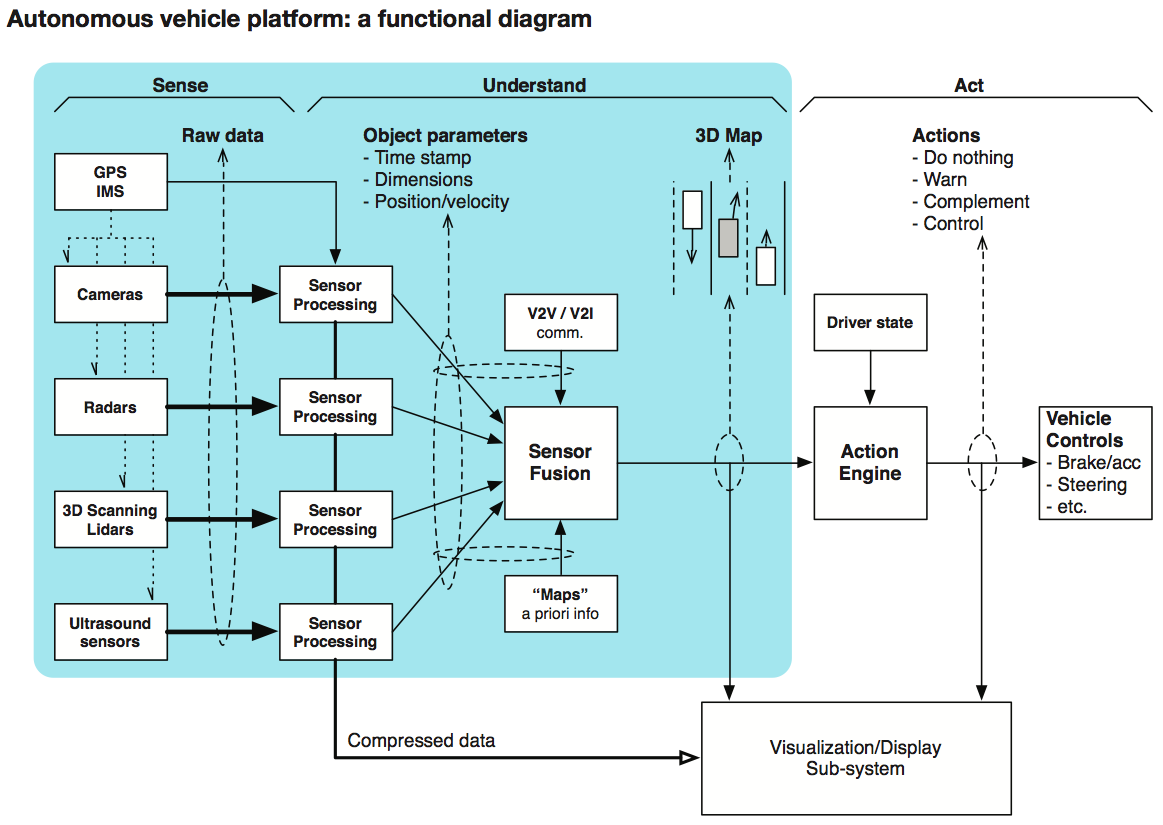